Mastering Machine Learning Data Annotation for Businesses
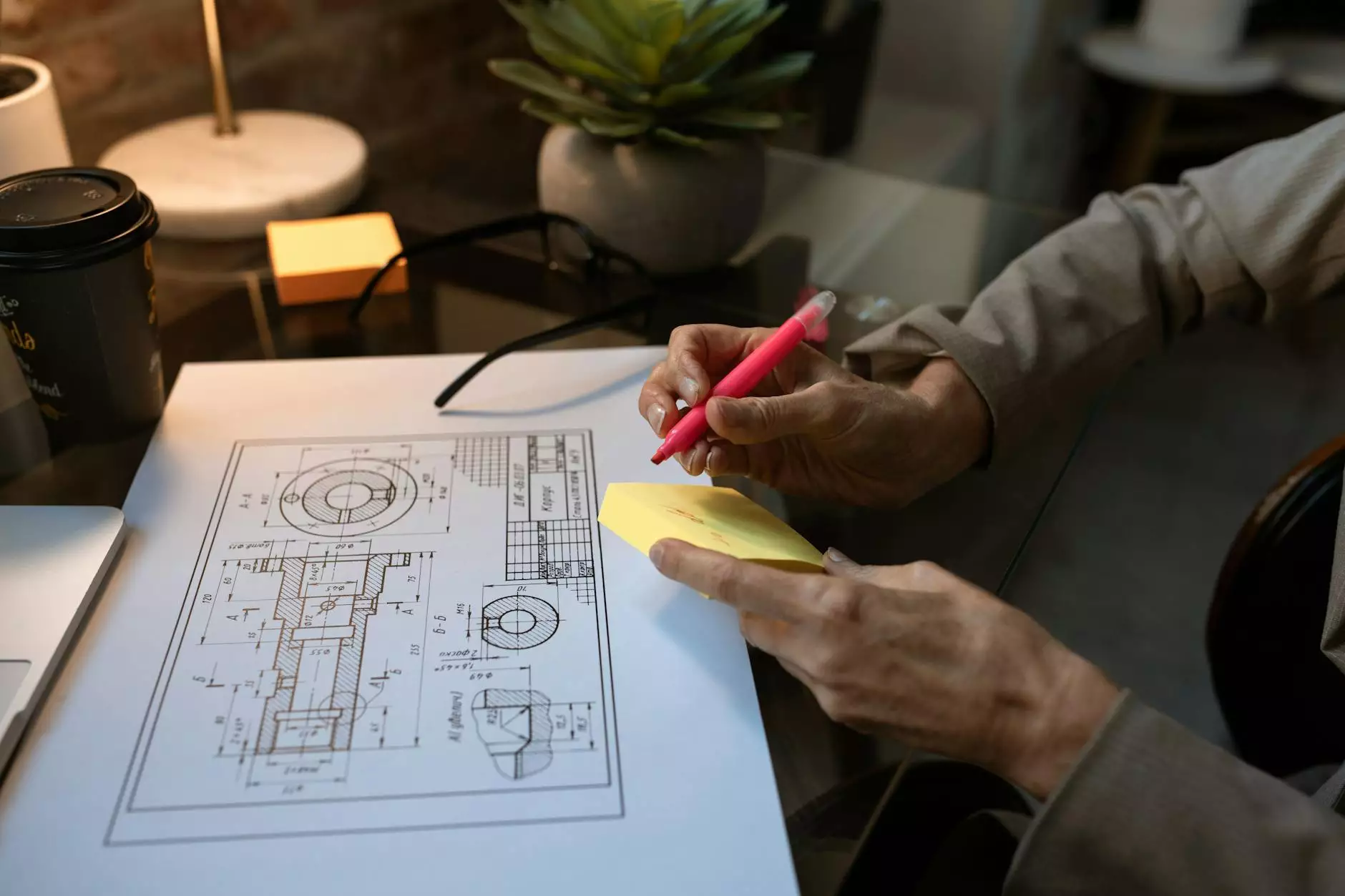
In today's fast-paced digital world, the way businesses operate is undergoing a significant transformation. Among the many technologies driving this change, machine learning stands out as a game-changer. However, the success of machine learning models heavily relies on one crucial aspect: data annotation. This article delves into the importance of machine learning data annotation, particularly in the context of home services and locksmith industries, helping businesses understand its impact on operational efficiency and customer satisfaction.
What is Machine Learning Data Annotation?
Machine learning data annotation refers to the process of labeling and tagging data to provide context for machine learning algorithms. This step is essential that allows computers to "understand" the data they process. Without properly annotated data, machine learning models cannot learn from the examples provided, leading to subpar performance and inaccurate predictions.
The Process of Data Annotation
Data annotation can take various forms depending on the type of data being used:
- Text Annotation: Labeling parts of text data to enhance understanding, such as tagging sentiment or identifying named entities.
- Image Annotation: Labeling images for tasks like object detection or image segmentation.
- Audio Annotation: Tagging audio files, often used in speech recognition and transcription tasks.
- Video Annotation: Labeling frames in video data for action recognition and surveillance.
Why Machine Learning Data Annotation Matters for Businesses
Data annotation is not just a technical requirement; it holds substantial implications for business success. Here are several reasons why machine learning data annotation is critical for businesses, especially in the home services and locksmith sectors:
1. Enhanced Operational Efficiency
By integrating machine learning models that rely on well-annotated data, businesses can automate various processes. For instance:
- Predictive Maintenance: Annotated data can predict when equipment might fail, allowing home service companies to schedule maintenance before issues arise.
- Customer Insights: Annotated consumer data helps in predicting customer behavior, enabling locksmiths to optimize their offerings and improve service delivery.
2. Improved Customer Satisfaction
Machine learning models trained on accurate and comprehensive data can personalize customer experiences. For example:
- By analyzing past service history, a locksmith business can offer tailored solutions to repeat customers.
- Home service providers can anticipate client needs based on annotated feedback data, allowing proactive adjustments to services.
3. Competitive Advantage
In an industry as competitive as home services and locksmiths, leveraging machine learning data annotation gives businesses an edge. Companies that harness the power of data effectively can:
- Optimize marketing strategies to target the right audience.
- Create data-driven business models that cater to evolving customer needs.
Effective Strategies for Implementing Data Annotation
For businesses looking to implement machine learning data annotation, consider the following strategies:
1. Define Clear Objectives
Before starting the data annotation process, it's vital to outline what you aim to achieve. Whether you want to improve customer service, streamline operations, or develop new products, having clear objectives will direct your data annotation efforts.
2. Invest in Quality Annotation Tools
Utilizing the right tools will streamline the data annotation process. Many tools can help automate aspects of annotation while ensuring high levels of accuracy, such as:
- Labelbox: A collaborative data labeling platform that ensures high-quality annotations.
- SuperAnnotate: Focuses on image and video annotations, particularly useful for visual data analysis.
- Prodigy: A machine learning annotation tool that allows for efficient text classification and integration with machine learning workflows.
3. Leverage Expert Annotators
While automated tools are beneficial, using human annotators can significantly enhance data quality. Skilled annotators can understand nuances and context that algorithms sometimes miss. This is especially crucial in industries like locksmithing, where service context can greatly influence customer needs.
4. Continuous Review and Improvement
Machine learning is an iterative process. After deploying models trained on annotated data, gather feedback and performance metrics. Use these insights to continually improve your annotations and model training processes, ensuring that they remain aligned with current business goals.
Challenges in Data Annotation and How to Overcome Them
While machine learning data annotation offers numerous benefits, businesses also face challenges, including:
1. High Costs
High-quality data annotation can be expensive, particularly if it requires hiring experts. To mitigate costs:
- Consider utilizing a crowdsourced annotation platform to lower costs while maintaining quality.
- Implement internal training programs for existing employees to develop annotation skills.
2. Time Constraints
Data annotation is often time-consuming. To address this issue:
- Use batch processing techniques to annotate data more quickly.
- Establish clear guidelines and workflows to speed up the annotation process.
3. Ensuring Quality and Consistency
Maintaining quality across annotated datasets can be challenging. To ensure consistency:
- Implement a benchmarking system to periodically evaluate annotation quality.
- Use a combination of automated techniques and human checks to enhance accuracy.
Conclusion: The Future of Machine Learning Data Annotation for Businesses
The role of machine learning data annotation in business is set to expand as industries continue to embrace technological advancements. For home service providers and locksmiths, adopting a robust data annotation strategy can lead to not just improved operational efficiency but also a profound transformation in the way they interact with customers. By incorporating the strategies outlined in this article, businesses can harness the full potential of machine learning, ensuring long-term growth and success.
Ultimately, as your business navigates the complexities of modern technology, remember that machine learning data annotation is not merely a checkbox on a project plan; it is a fundamental element that can propel your business into the future. Invest in quality data annotation today for a smarter, more efficient tomorrow.